Most of my waking hours are now dedicated to AI, with an occasional nap in between. This is the first in a series of regular pieces where I share my ongoing learnings and thoughts. I keep these short and sweet—under 3 minutes—because who has time for a 3,000-word article when you could be binge-watching cat videos?
Several weeks ago, I stumbled upon the ARC Prize, a $1M+ competition funded by Zapier co-founder Mike Knoop and AI researcher François Chollet. The challenge? Get an AI model to surpass an 85% score on their reasoning test.

Despite the best efforts from the brightest minds on the planet, the highest AI score so far is just 39%, whereas an average person could easily score 80%. Humans: 1, AI: 0.
The race to AGI has always been a hot topic. Some visionaries and researchers (e.g. Ray Kurzweil, Leopold Aschenbrenner) are highly optimistic, predicting we’ll hit Artificial General Intelligence (AGI) by 2027 - 2029.
But the big question remains: What does it even mean to be intelligent?
What Makes Intelligence, Well, Intelligent?
Here’s a hot take: A newborn baby is 10X more intelligent than GPT-4.
Why? Babies are curiosity machines. They mimic sounds, copy actions, remember faces, and understand emotions. They explore their world with a relentless drive to learn. Through play and interaction, they soak up knowledge and learn new skills at an astonishing rate.
You know, babies understand the world much better than we think:
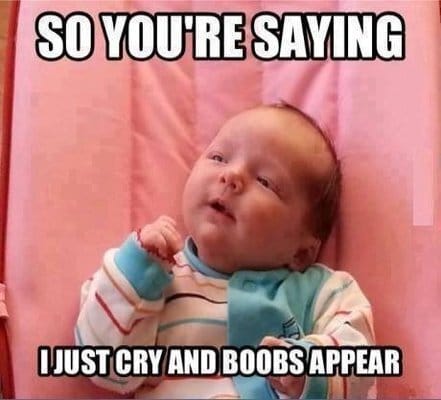
Smart baby. Source: Babycenter.ca
Sure, it’s easy to be wowed by AI today. AI can code, create stunning art, plan vacations, and even produce Netflix-worthy TV shows. Headlines scream about AI beating human doctors at medical licensing exams.
These are impressive. But they are also noise.
These are things we have already accomplished as humans or knowledge we have already acquired. They can be learned with enough effort and time—or, in AI terms, with sufficient compute and data
Today’s large language models (LLMs) are sophisticated heuristic engines. They pull from vast databases of information to deliver what they think is the best answer.
While this often works well, it lacks the depth and deliberation of true analytical thinking. Some models are beginning to exhibit early forms of reasoning and problem-solving, which is heartening.
The hallmark of generalised intelligence is the ability to learn and adapt to new situations.
And that’s why a baby, with its insatiable curiosity, trumps GPT-4 on intelligence (though not in knowledge).
The Road to AGI
So, how do we get to AGI? The roadmap is murky, and I’m not betting on it happening this decade.
We’ll need groundbreaking new architectures, much more compute power ($1 trillion training clusters?), and higher quality datasets—just for starters.
Achieving AGI will require stepping beyond the confines of current deep learning paradigms. We must blend in principles from symbolic reasoning and cognitive architectures that mimic human thinking patterns and pull in interdisciplinary insights from cognitive science.
Allowing models to learn through human interaction and feedback will enhance their adaptability and reasoning capabilities. Integrating cognitive skills such as attention, memory, and planning into AI models can make them more adept at reasoning and problem-solving.
We’re on a slow but steady journey toward true generalised intelligence. The story is still being written, and it’s bound to be a page-turner.
Cheers,

